Navigating the AI assets management Landscape: A Practical Guide
Your Roadmap to Seamless Integration
The landscape of asset management is rapidly evolving, and at the forefront of this transformation is artificial intelligence (AI). AI's ability to process vast amounts of data, identify patterns, and make informed decisions has revolutionized the financial industry. However, navigating the AI assets management landscape can be complex. This practical guide aims to provide a comprehensive understanding of AI in asset management, including its benefits, challenges, and best practices for implementation.
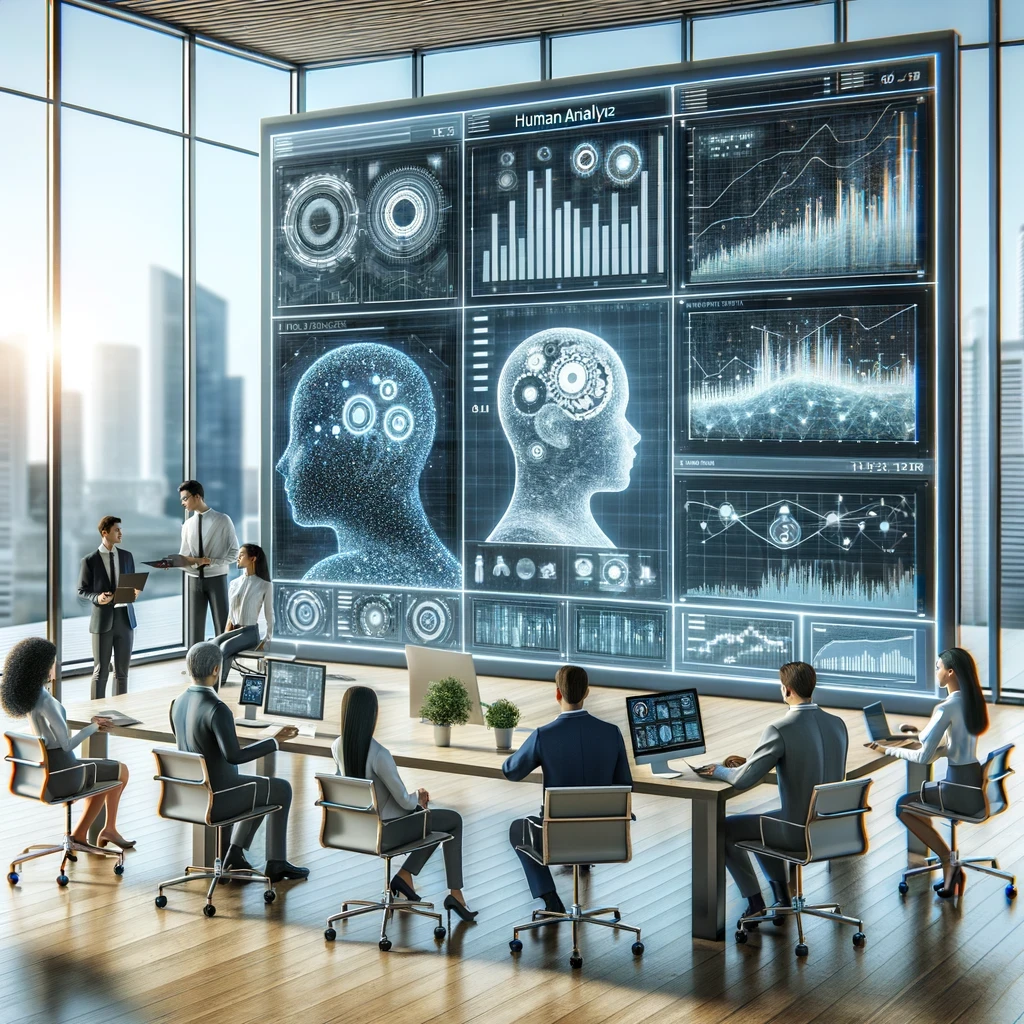
Understanding AI in Asset Management
AI in asset management refers to the use of machine learning algorithms, natural language processing, and other AI technologies to analyze financial data, predict market trends, and optimize investment strategies. AI can enhance portfolio management, risk assessment, and trading by providing insights that are beyond human capabilities.
Key Components of AI in Asset Management:
1. Machine Learning (ML): Algorithms that learn from historical data to predict future market behavior.
2. Natural Language Processing (NLP): Techniques that analyze and interpret human language, useful for sentiment analysis and news interpretation.
3. Robotic Process Automation (RPA): Automation of repetitive tasks, improving efficiency and reducing errors.
4. Predictive Analytics: Using statistical models to forecast market trends and asset performance.
Benefits of AI in Asset Management
AI offers numerous benefits that can significantly enhance asset management practices:
1. Improved Decision Making: AI can analyze vast datasets quickly and accurately, providing insights that help in making informed investment decisions.
2. Increased Efficiency: Automation of routine tasks allows asset managers to focus on strategic decision-making, improving overall efficiency.
3. Enhanced Risk Management: AI can identify potential risks by analyzing market trends and historical data, enabling proactive risk mitigation.
4. Cost Reduction: By automating processes and improving accuracy, AI can reduce operational costs associated with asset management.
5. Personalized Investment Strategies: AI can tailor investment strategies to individual investor profiles, enhancing client satisfaction and retention.
Challenges of Implementing AI in Asset Management
While AI presents many opportunities, it also comes with challenges that need to be addressed:
1. Data Quality and Availability: High-quality, comprehensive data is crucial for effective AI models. Incomplete or inaccurate data can lead to poor decision-making.
2. Complexity and Expertise: Implementing AI requires specialized knowledge and expertise, which may be lacking in traditional asset management firms.
3. Regulatory Compliance: Navigating the regulatory landscape is challenging, as AI technologies must comply with existing financial regulations.
4. Ethical Considerations: Ensuring AI systems are fair, transparent, and unbiased is essential to maintain trust and integrity in asset management.
5. Integration with Existing Systems: AI solutions must be seamlessly integrated with existing IT infrastructure, which can be complex and costly.
Best Practices for Implementing AI in Asset Management
To successfully implement AI in asset management, firms should follow these best practices:
Start with a Clear Strategy:
- Define the goals and objectives for AI implementation.
- Identify specific areas where AI can add value, such as portfolio management, risk assessment, or customer service.
- Develop a roadmap for AI integration, including timelines, resources, and key milestones.
Invest in Data Management:
- Ensure access to high-quality, relevant data.
- Implement robust data management practices to maintain data integrity and accuracy.
- Use data augmentation techniques to enhance datasets where necessary.
Build a Skilled Team:
- Recruit or train staff with expertise in AI, data science, and finance.
- Foster a culture of continuous learning to keep up with advancements in AI technologies.
- Encourage collaboration between data scientists and financial experts to develop effective AI models.
Ensure Regulatory Compliance:
- Stay informed about regulatory developments related to AI and finance.
- Implement compliance checks and audits to ensure AI systems adhere to relevant regulations.
- Work with legal experts to navigate complex regulatory requirements.
Focus on Ethical AI:
- Develop AI systems that are transparent and explainable.
- Conduct regular audits to identify and mitigate biases in AI models.
- Implement ethical guidelines to govern the use of AI in asset management.
Invest in Technology Infrastructure:
- Ensure that the IT infrastructure can support AI workloads.
- Use cloud-based solutions to scale AI capabilities as needed.
- Implement robust cybersecurity measures to protect data and AI systems from threats.
Measure and Monitor Performance:
- Establish key performance indicators (KPIs) to measure the success of AI initiatives.
- Use real-time monitoring to track AI performance and make necessary adjustments.
- Regularly review and update AI models to ensure they remain effective and relevant.
Real-World Applications of AI in Asset Management
To understand the practical implications of AI in asset management, consider the following real-world applications:
1. Algorithmic Trading: AI algorithms can analyze market data and execute trades at high speeds, capitalizing on market inefficiencies and improving returns.
2. Portfolio Optimization: AI can assess various factors such as risk tolerance, market conditions, and historical performance to optimize investment portfolios.
3. Predictive Maintenance: For asset-heavy industries, AI can predict equipment failures and optimize maintenance schedules, reducing downtime and costs.
4. Sentiment Analysis: AI can analyze news articles, social media, and other sources to gauge market sentiment, informing investment strategies.
5. Client Advisory: AI-driven robo-advisors can provide personalized investment advice based on individual client profiles and market conditions.
Case Study: Successful AI Implementation
Case Study: Treem Finance
Treem Finance, a mid-sized firm, decided to integrate AI into their operations to enhance portfolio management and risk assessment. Here's how they did it:
Step 1: Strategy Development
- Treem Finance identified portfolio optimization and risk management as key areas for AI implementation.
- They set clear objectives: improve portfolio returns by 10% for conservative funds and reduce risk by 15% over two years.
Step 2: Data Management
- The firm invested in a data management system to ensure access to high-quality financial data.
- They partnered with data providers to augment their datasets with real-time market data.
Step 3: Building a Skilled Team
- Treem Finance hired data scientists and AI experts to work alongside their financial analysts.
- They provided ongoing training to ensure the team stayed updated on AI advancements.
Step 4: Regulatory Compliance
- The firm worked with legal experts to ensure their AI systems complied with financial regulations.
- They conducted regular compliance audits to maintain adherence to regulatory standards.
Step 5: Ethical AI
- Treem Finance developed ethical guidelines for AI use, focusing on transparency and fairness.
- They implemented regular bias audits to ensure their AI models were unbiased and equitable.
Step 6: Technology Infrastructure
- The firm upgraded their IT infrastructure to support AI workloads, using cloud-based solutions for scalability.
- They implemented robust cybersecurity measures to protect their data and AI systems.
Step 7: Performance Measurement
- Treem Finance established KPIs to measure the success of their AI initiatives.
- They used real-time monitoring to track AI performance and made adjustments as needed.
Results:
- Within two years, Alpha Asset Management improved their portfolio returns by 12% and reduced risk by 18%.
- Their AI-driven strategies provided valuable insights, enhancing decision-making and operational efficiency.
Future Trends in AI Asset Management
The future of AI in asset management looks promising, with several emerging trends set to shape the industry:
1. AI and Blockchain Integration: Combining AI with blockchain technology can enhance transparency, security, and efficiency in asset management.
2. Advanced Predictive Analytics: Improved AI algorithms will provide even more accurate market predictions, helping asset managers make better-informed decisions.
3. AI-Powered ESG Investing: AI can analyze environmental, social, and governance (ESG) factors, enabling asset managers to make more sustainable investment choices.
4. Personalized Investment Solutions: AI will continue to evolve, offering even more personalized investment strategies tailored to individual investor profiles.
Navigating the AI assets management landscape requires a strategic approach, balancing innovation with ethical considerations. By understanding the benefits, addressing the challenges, and following best practices, asset managers can harness the power of AI to enhance decision-making, improve efficiency, and deliver better returns for their clients. As AI technology continues to advance, staying informed and adaptable will be key to thriving in this dynamic industry.